The Discipline and Future of Machine Learning
Over the past 50 years the study of machine learning has grown from the efforts of a handful of computer engineers exploring whether computers could learn to play games, and a field of statistics that largely ignored computational considerations, to a broad discipline that has produced fundamental statistical-computational theories of learning processes, has designed learning algorithms that are routinely used in commercial systems from speech recognition to computer vision, and has spun off an industry in data mining to discover hidden regularities in the growing volume of online data.
This talk will provide a personal view of the current state of machine learning, and where I think the field might (should) be headed over the coming decade. I’ll propose several specific research areas which seem to me to have great potential, and will leave plenty of time at the end for audience discussion.
Speaker Bio
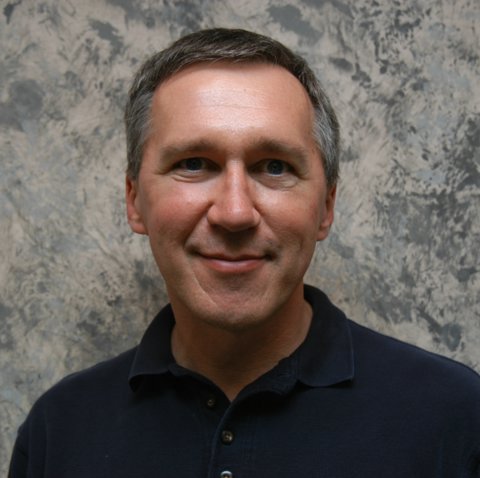
Tom M. Mitchell is the E. Fredkin Professor and head of the Machine Learning Department at Carnegie Mellon University. He is Chair of the American Association for the Advancement of Science (AAAS) section on Information, Computing and Communication, Past President of the American Association of Artificial Intelligence (AAAI), and a recent member of the US National Research Council's Computer Science and Telecommunications Board. His current research projects include machine learning approaches to modeling human brain function using fMRI and behavioral data, and statistical machine learning approaches to reading the web automatically. Mitchell believes the field of machine learning will be the fastest growing part of computer science during the next 25 years.