Binge Watching: Scaling Affordance Learning from Sitcoms
|
|
|

Abstract
In recent years, there has been a renewed interest in jointly modeling perception and action. At the core of this investigation is the idea of modeling affordances (Affordances are opportunities of interaction in the scene. In other words, it represents what actions can the object be used for). However, when it comes to predicting affordances, even the state of the art approaches still do not use any ConvNets. Why is that? Unlike semantic or 3D tasks, there still does not exist any large-scale dataset for affordances. In this paper, we tackle the challenge of creating one of the biggest dataset for learning affordances. We use seven sitcoms to extract a diverse set of scenes and how actors interact with different objects in the scenes. Our dataset consists of more than 10K scenes and 28K ways humans can interact with these 10K images. We also propose a two-step approach to predict affordances in a new scene. In the first step, given a location in the scene we classify which of the 30 pose classes is the likely affordance pose. Given the pose class and the scene, we then use a Variational Autoencoder (VAE) to extract the scale and deformation of the pose. The VAE allows us to sample the distribution of possible poses at test time. Finally, we show the importance of large-scale data in learning a generalizable and robust model of affordances.
Paper and Dataset
![]() |
Xiaolong Wang*, Rohit Girdhar* and Abhinav Gupta Binge Watching: Scaling Affordance Learning from Sitcoms Proc. of IEEE Conference on Computer Vision and Pattern Recognition (CVPR), 2017 (*indicates equal contribution.) [PDF] [dataset] [pose cluster centers] |
CVPR Spotlight Talk
Poses Predicted by Our Model
Pose predicted by our model on FRIENDS TV Shows.

Sampling Different Poses in the Same Location
We use VAE to model the uncertainty of poses, we can sample different reasonable results on the same location.
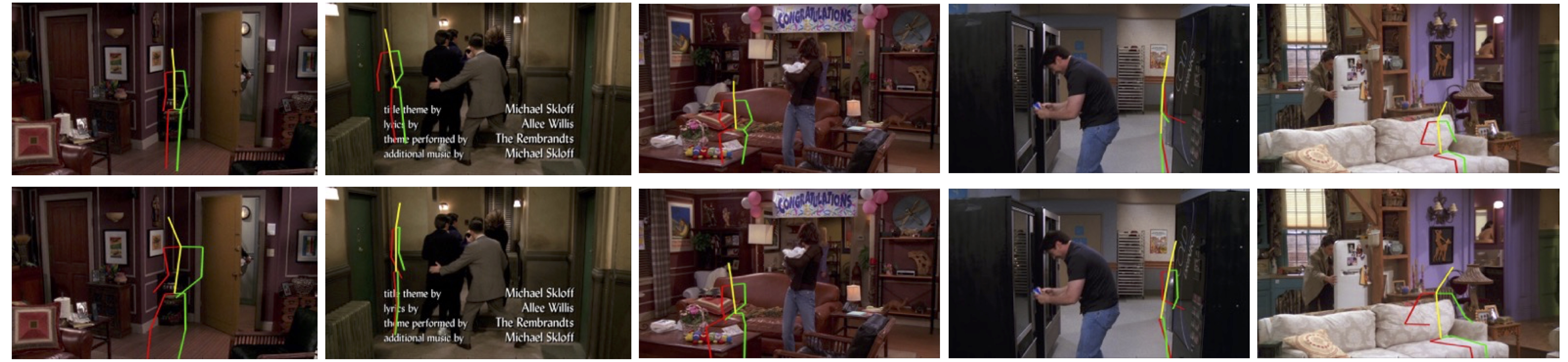
Generalization to NYUv2
We apply our trained model on the NYUv2 test set as shown below. We can see that although our model is learned in TV show videos, it can generate very reasonable results in NYU dataset. For example, the second pose in the first row is sitting beside the minibar.
